We are team of technical nerds, Hire Us for your next project
3D Point Cloud Annotation Services
- Home
- 3D Point Cloud Annotation Services
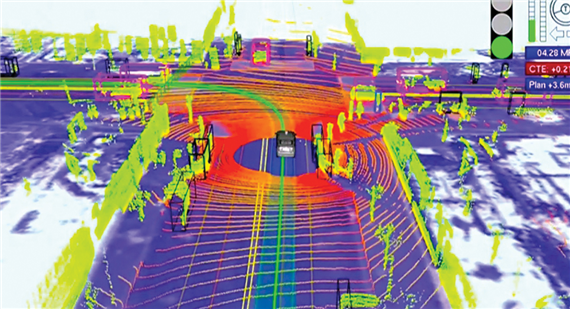
//Video annotation
3D Point Cloud Annotation
3D point cloud annotation is a crucial process in computer vision and machine learning, particularly in applications such as autonomous driving, robotics, augmented reality, and urban planning. Point clouds are three-dimensional datasets composed of numerous points in space, typically captured by sensors like LiDAR or depth cameras. Annotating these point clouds involves labeling individual points or groups of points to provide semantic meaning or object classification
//3D POINT CLOUD ANNOTATION
APPLICATIONS OF 3D POINT CLOUD ANNOTATION
Object Detection and Recognition
Annotating 3D point clouds involves labeling objects of interest within the scene. This can include vehicles, pedestrians, cyclists, buildings, road signs, and other relevant entities. By labeling these objects, machine learning models can be trained to detect and recognize them in real-world scenarios, crucial for applications like autonomous driving and robotics.
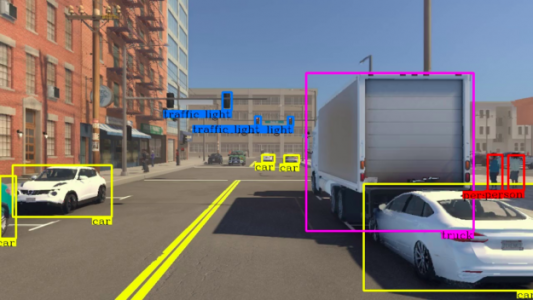
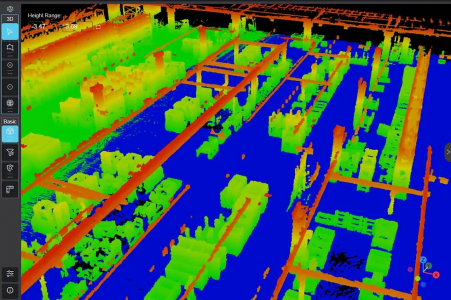
Semantic Segmentation
Similar to image segmentation, semantic segmentation in 3D point clouds involves partitioning the scene into semantically meaningful regions or segments. Each point or group of points is assigned a semantic label corresponding to a specific object class or category. Semantic segmentation enables a finer understanding of the scene's composition and facilitates tasks such as scene understanding and reconstruction.
Instance Segmentation
Instance segmentation in 3D point clouds involves not only labeling points with semantic categories but also distinguishing between individual object instances. This is particularly important in scenarios with multiple instances of the same object class, such as multiple vehicles or pedestrians in a scene. Instance segmentation enables precise localization and tracking of individual objects.
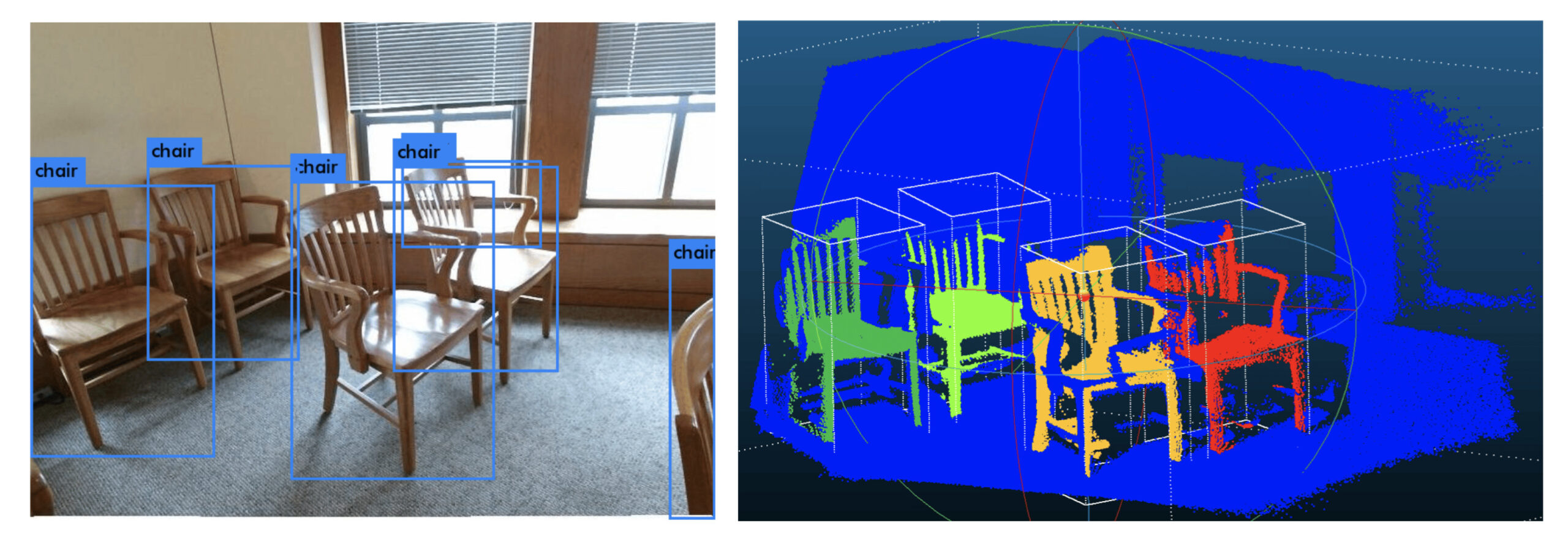
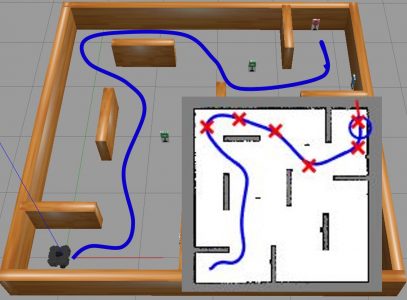
Obstacle Detection and Avoidance
Annotating point clouds with obstacle labels helps autonomous systems detect and avoid potential obstacles in their environment. By accurately labeling points representing obstacles such as walls, barriers, or debris, autonomous vehicles and robots can plan safe trajectories and navigate complex environments.
LiDAR Annotation for Autonomous Vehicles
LiDAR sensors generate dense point clouds that provide rich information about the surrounding environment. 3D point cloud annotation is essential for annotating LiDAR data to train machine learning models for autonomous driving tasks such as object detection, localization, and path planning. Annotated LiDAR data helps autonomous vehicles perceive and navigate complex environments safely.
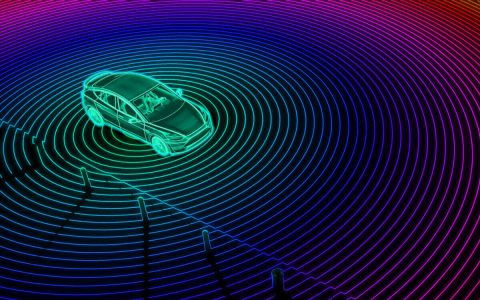
//Industries
USE CASES FOR 3D POINT CLOUD ANNOTATION
RETAIL
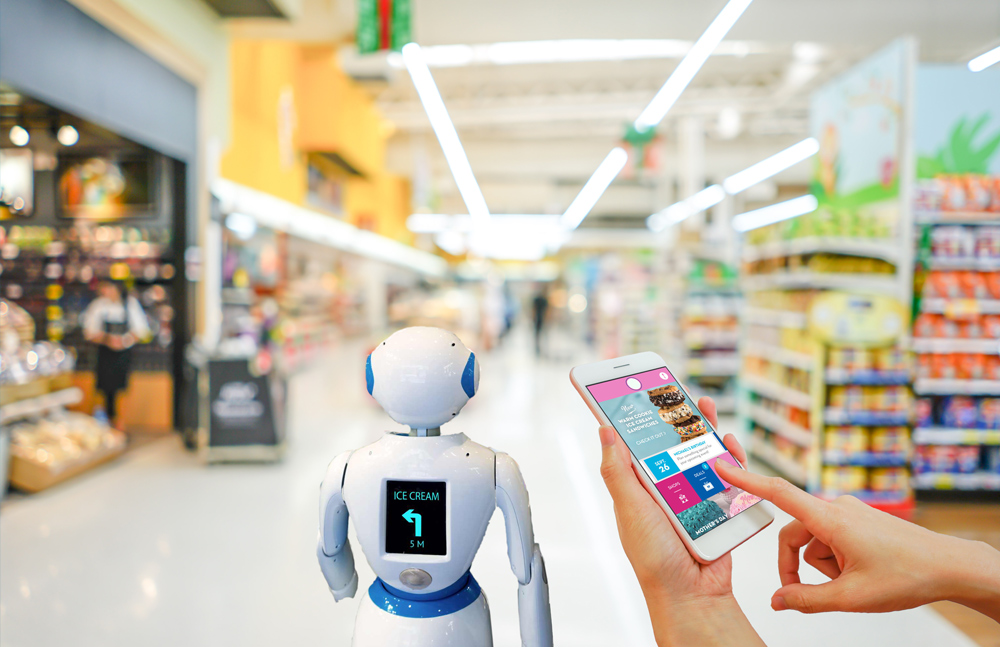
ROBOTICS
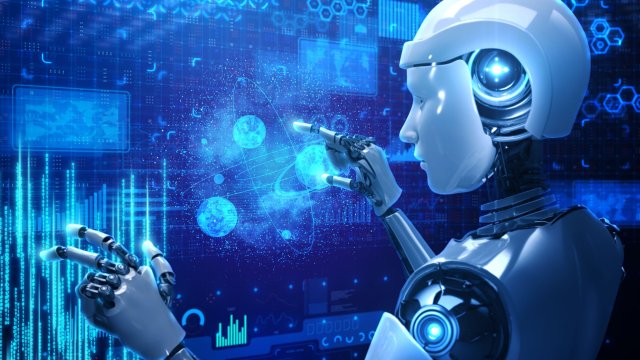
AGRICULTURE
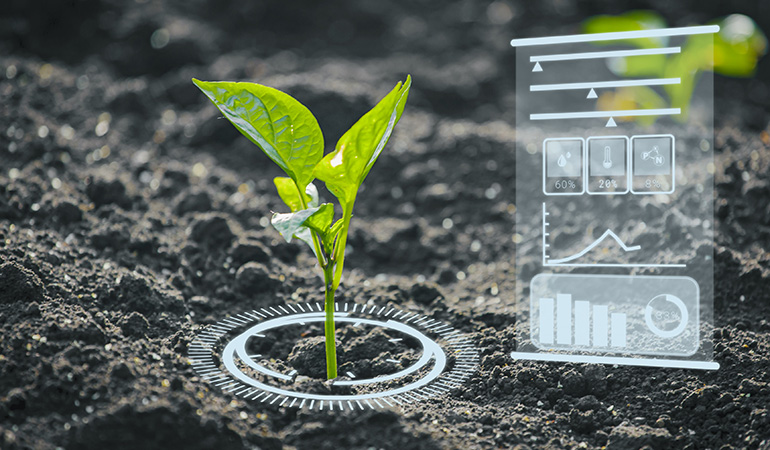
INSURANCE
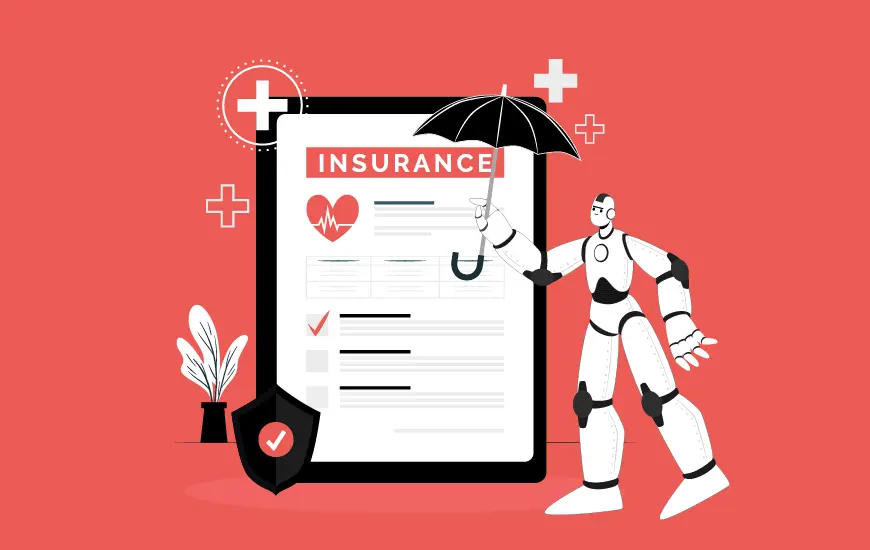
HEALTHCARE
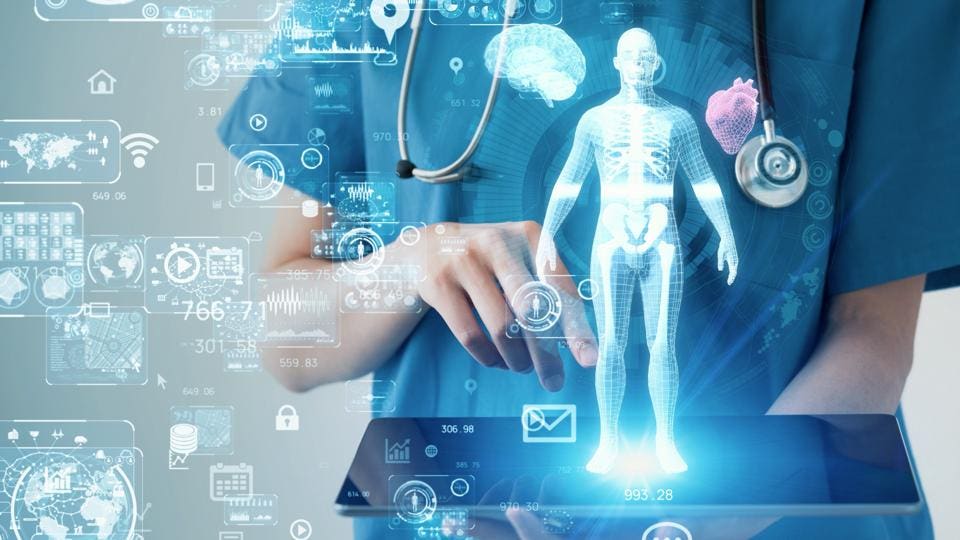
SECURITY & SURVEILLANCE
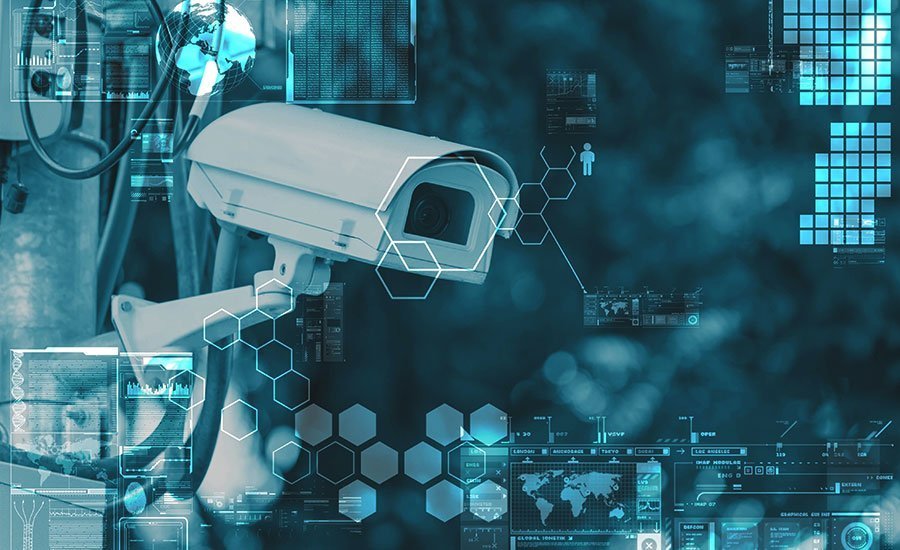
SELF-DRIVING
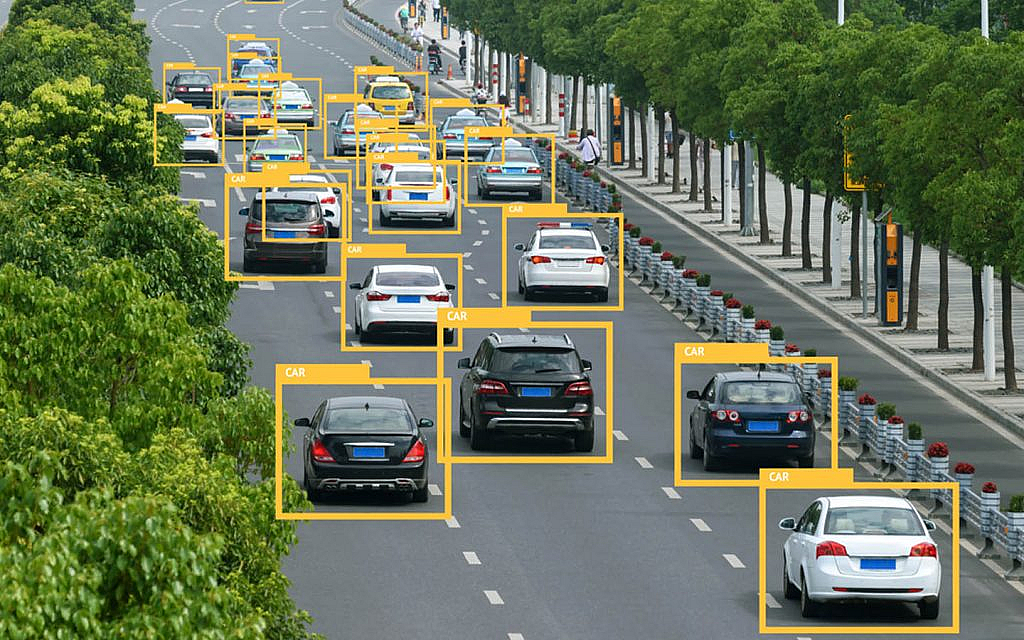
LOGISTICS
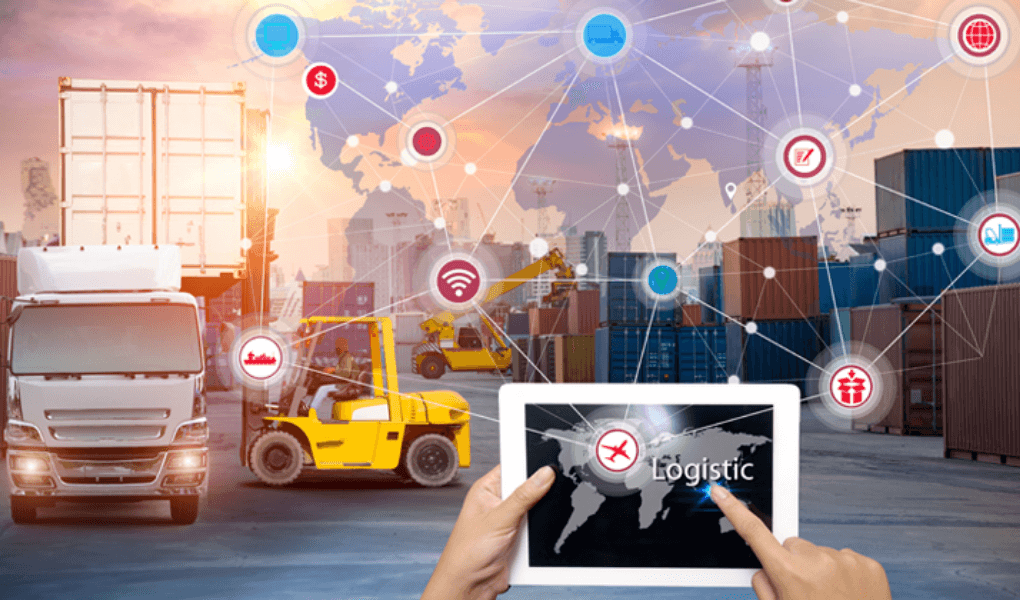
AUTONOMOUS FLYING
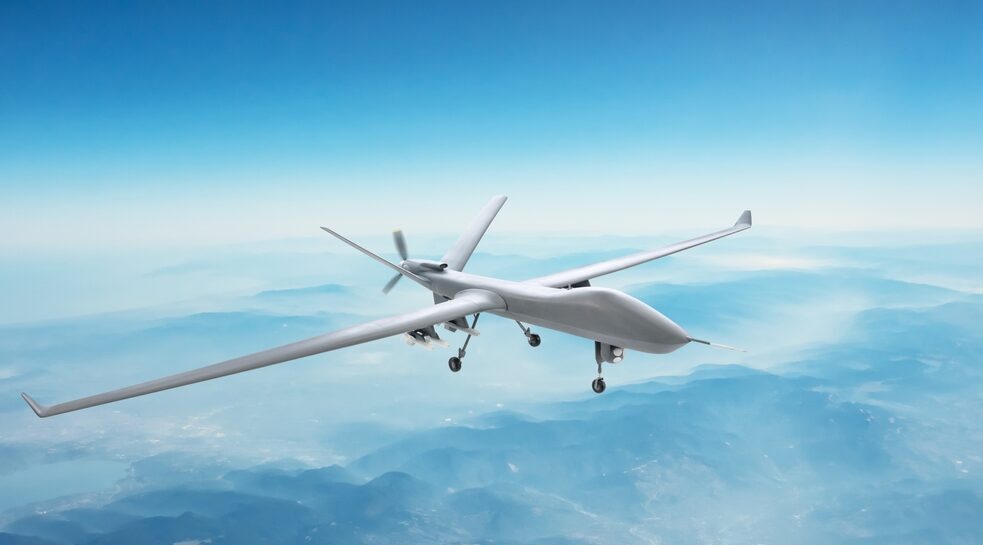
// Drop us a line! We are here to answer your queries